Cohort Metrics For Startups Revealed – Part II: Aged Groups
500 Startups Mentor Dan Martell (@danmartell) continues with Part 2 of “Cohort Metrics for Startups Revealed.” Catch up on Part 1 here.
Authors note: This post was written in collaboration with Assaf Arkin, Flowtown’s lead engineer and the creator of Vanity – a ruby framework for experiment driven development (EDD).
In part one we reviewed segmenting customers into cohorts, understanding what channel they were acquired through and then looked at their behavior over time. That was the easy part. The cohort analysis that throws a curve ball for most people is the one dealing with age groups. And no, we’re not talking about the biological age of your customers, but how long they’ve been a customer.
Weekly cohorts
Let’s say we have a service, BackToPlain, that undoes any filters applied to Instagram photos. We’re interested in looking at active customers. Active customers engage in some behavior that correlates with value to them, in our case, uploading and un-filtering images.
So the first and easiest number we can tackle is the number of active users this week. That one is easy to measure, it’s a simple count. We see that it’s growing 5% week over week.
The second number we’re going to look for is conversion, in our case the percentage of users activated. Effectively we’re calculating the ratio of users who, at some point, became active. It’s a valueable number: if our product is too technical or just difficult to use, that number will be low. If it provides a lot of immediate value and feedback, it will be high.
It’s also a slighly misleading number. It turns out our activation rate is 30%, which is not a bad number, but let’s work the rest of them. It costs us $85 to acquire a user, 35% activate, each user pays $10 a month (or we make the equivalent some other way). Are we profitable?
Well, that depends on what we call the Life Time Value of each of these users, which depends on how much they’re paying a month, and how long they’re sticking around.
Activation doesn’t tell us that, it shows that 35% of users were active at some point in time (converted), but maybe they were only active for a week and promptly left. The number of active users keep going up 5% week over week, but what if we’re activating 300 new users but losing 250. What if the average user sticks around for two months, paying $20 in total. Remember, that user cost $85 to acquire.
This is where we need to understand behavior over time, but we can’t look at aggregate. An aggregate would look something like:
[table id=3 /]
What instead we need to look at, is a table like this:
[table id=6 /]
The weeks here refer to when the user signed up, so we see that 350 users are active in the first seven days after they sign up. In the next seven days, 300 of these remain active. In the fifth week after signing up, that’s the second month, only 50 remain active.
Basically, we get users to toy around for a couple of weeks, then they lose interest and only 14% stick around for the second month of service. That could be good enough if we didn’t pay so much to acquire users.
Of course, that chart may not be the most accurate either. What if those 50 are the friends and family we invited early on, meaning we really don’t have retention with the general population? Or maybe our product is getting better? Of the 100 that joined this month, 50 will stick around. It’s the numbers from earlier months, when we had less features and more service interruptions, that are confusing us.
So let’s change the table a bit:
[table id=4 /]
The horizontal are cohorts: they’re grouped by users who joined the service on a particular week. The top row represents all the users who joined the week of June 5th, the next row are users who joined the week of June 12th and so forth.
For each cohort we see a time series of the weekly active users. The week here refers to their week of being with the service, so the top left would be users who joined on the week of June 5th, 100 of which were active during the week of June 5th.
Of these 100, only 20 remained active in their second week of using the service, the week of June 12th. Note that in that week, 100 other users joined the service; those are represented in the second row. The first row only deals with users who joined the week of the 5th. Those who were really not happy in their first week chose not to stick around for long.
But something interesting happened in the week of June 12th. We made a significant improvement to the service, and even though we lost a lot of users that came the previous week (before these changes), we retained 40 that came that week and experienced those changes. 30 of them stuck around for four weeks.
Those users who joined the week of June 19th, after the new changes were rolled out and some bugs ironed out, were even happier. 50 stuck around for their second week of service.
So we’re making a significant improvement to the service that we know. Our second week retention went from 20 to 50, 150% improvement; our second week retention went from 15 to 44, up 190%.
In contrast, if we rolled up the data, we’d see a different picture:
[table id=5 /]
There’s some improvements, but it’s not as spectacular because the roll-up swallows significant change in historical information.
It’s cohorts that allow us to clearly see any significant improvement or failure in real time and respond to it.
If you have any questions, please don’t hesitate to leave a question in the comments.
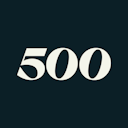
500 Global Team